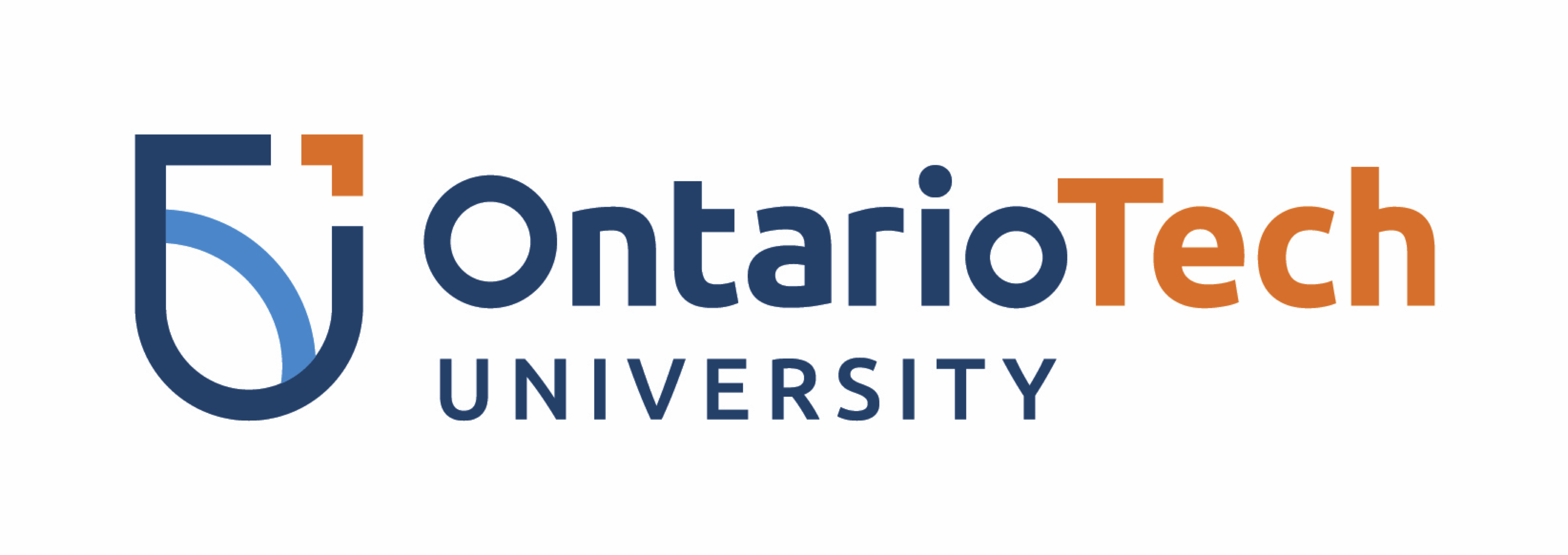
Recurrent Neural Networks¶
Faisal Z Qureshi
faisal.qureshi@ontariotechu.ca
http://www.vclab.ca
Credits¶
These slides draw heavily upon works of many individuals, notably among them are:
- Nando de Freitas
- Fei-Fei Li
- Andrej Karpathy
- Justin Johnson
Lesson Plan¶
- Sequential processing of fixed inputs
- Recurrent neural networks
- LSTM
Idea¶
- Sequential processing of fixed inputs
$\newcommand{\bh}{\mathbf{h}}$
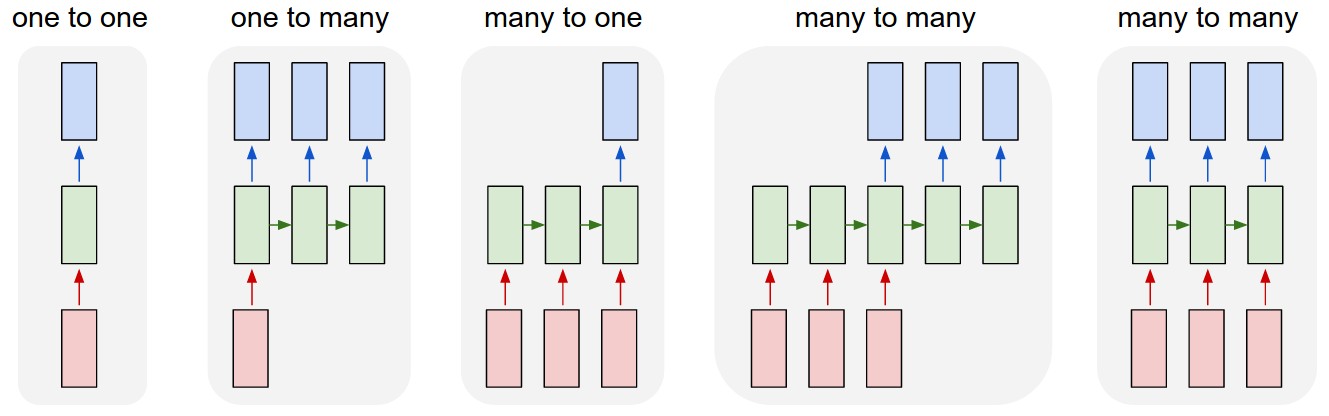
- one to one: image classification
- one to many: image captioning
- many to one: sentiment analysis
- many to many: machine translation
- many to many: video understanding
[From A. Karpathy Blog]
$\newcommand{\bx}{\mathbf{x}}$
Recurrent Neural Network¶
- $\bh_t = \phi_1 \left( \bh_{t-1}, \bx_t \right)$
- $\hat{y}_t = \phi_2 \left( \bh_t \right)$
Where
- $\bx_t$ = input at time $t$
- $\hat{y}_t$ = prediction at time $t$
- $\bh_t$ = new state
- $\bh_{t-1}$ = previous state
- $\phi_1$ and $\phi_2$ = functions with parameters $W$s that we want to train
Subscript $t$ indicates sequence index.
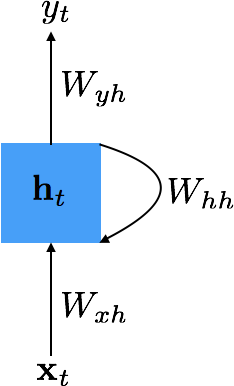
Example¶
$$ \begin{split} \bh_t &= \tanh \left( W_{hh} \bh_{t-1} + W_{xh} \bx_t \right) \\ \hat{y}_t &= \text{softmax} \left( W_{hy} \bh_t \right) \end{split} $$
Unrolling in Time¶
- Parameters $W_{xh}$, $W_{hh}$ and $W_{hy}$ are tied over time
- Cost: $E = \sum_t E_t$, where $E_t$ depends upon $y_t$
- Training: minimize $E$ to estimate $W_{xh}$, $W_{hh}$ and $W_{hy}$
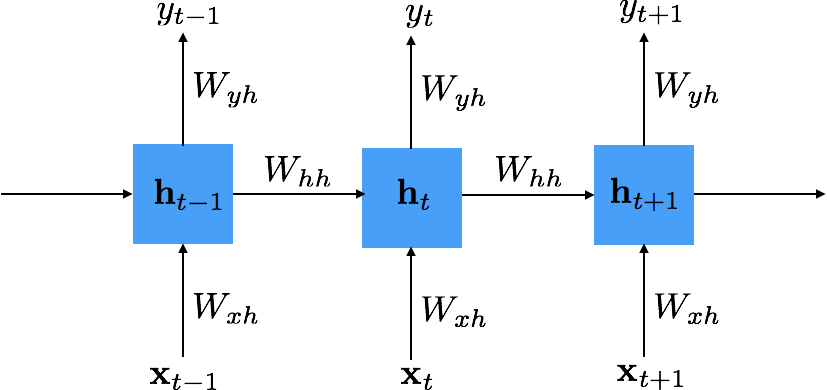
Loss¶
When dealing with output sequences, we can define loss to be a function of the predicted output $\hat{y}_t$ and the expected value $y_t$ over a range of times $t$
$$ E(y,\hat{y}) = \sum_t E_t(y, \hat{y}) $$
Example: using cross-entropy for k-class classification problem¶
$$ E(y,\hat{y}) = - \sum_t y_t \log \hat{y}_t $$
Computing Gradients¶
We need to compute $\frac{\partial E}{\partial W_{xh}}$, $\frac{\partial E}{\partial W_{hh}}$ and $\frac{\partial E}{\partial W_{hy}}$ in order to train an RNN
Example¶
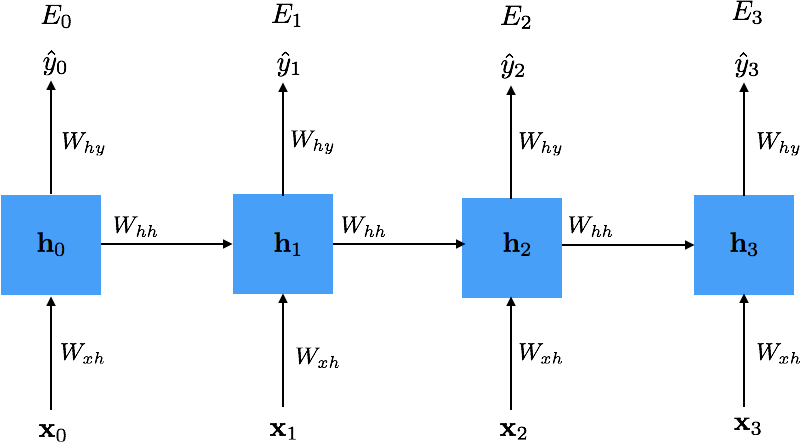
$$ \frac{\partial E_3}{\partial W_{hh}} = \sum_{k=0}^3 \frac{\partial E_3}{\partial \hat{y}_3} \frac{\partial \hat{y}_3}{\partial \bh_3} \frac{\partial \bh_3}{\partial \bh_k} \frac{\partial \bh_k}{\partial W_{hh}} $$
Vanishing and Exploding Gradients¶
We can compute the highlighted term in the following expression using chain-rule $$ \frac{\partial E_3}{\partial W_{hh}} = \sum_{k=0}^3 \frac{\partial E_3}{\partial \hat{y}_3} \frac{\partial \hat{y}_3}{\partial \bh_3} \color{red}{\frac{\partial \bh_3}{\partial \bh_k}} \frac{\partial \bh_k}{\partial W_{hh}} $$ Applying the chain-rule $$ \frac{\partial \bh_3}{\partial \bh_k} = \prod_{j=k+1}^3 \frac{\partial \bh_j}{\partial \bh_{j-1}} $$ Or more generally $$ \frac{\partial \bh_t}{\partial \bh_k} = \prod_{j=k+1}^t \frac{\partial \bh_j}{\partial \bh_{j-1}} $$
Difficulties in Training¶
$$ \frac{\partial E_t}{\partial W_{hh}} = \sum_{k=0}^t \frac{\partial E_t}{\partial \hat{y}_t} \frac{\partial \hat{y}_t}{\partial \bh_t} \left( \prod_{j=k+1}^t \frac{\partial \bh_j}{\partial \bh_{j-1}} \right) \frac{\partial \bh_k}{\partial W_{hh}} $$
$\frac{\partial \bh_i}{\partial \bh_{i-1}}$ is a Jacobian matrix.
For longer sequences
- if $\left| \frac{\partial \bh_i}{\partial \bh_{i-1}} \right| < 0$, the gradients vanish
- Gradient contributions from "far away" steps become zero, and the state at those steps doesn’t contribute to what you are learning.
- Long short-term memory units are designed to address this issue
- if $\left| \frac{\partial \bh_i}{\partial \bh_{i-1}} \right| > 0$, the gradients explode
- Clip gradients at a predefined threshold
- See also, On the difficulty of training recurrent neural networks, Pascanu et al.
Image Captioning Example¶
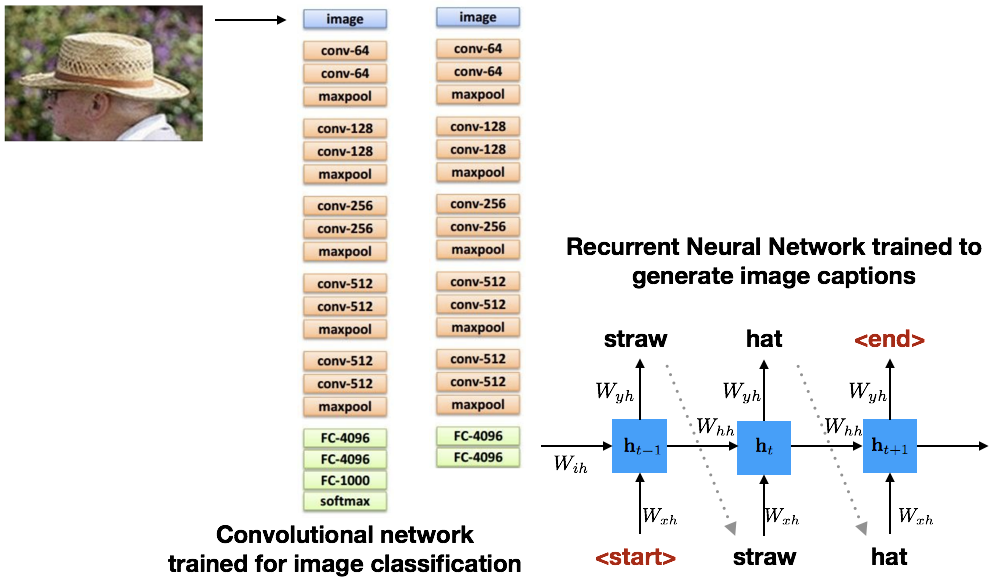
For the image captioning example shown in the previous slide, $\bh_t$ is defined as follows:
$$ \begin{split} \bh_t &= \tanh (W_{hh} \bh_{t-1} + W_{xh} \bx + \color{red}{W_{ih} \mathbf{v}}) \\ \hat{y}_t &= \text{softmax} (W_{hy} \bh_{t}) \end{split} $$
Image Captioning¶
- Explain Images with Multimodal Recurrent Neural Networks, Mao et al.
- Deep Visual-Semantic Alignments for Generating Image Descriptions, Karpathy - and Fei-Fei
- Show and Tell: A Neural Image Caption Generator, Vinyals et al.
- Long-term Recurrent Convolutional Networks for Visual Recognition and Description, Donahue et al.
- Learning a Recurrent Visual Representation for Image Caption Generation, Chen and Zitnick
Dealing with Vanishing Gradients¶
Change of notation.$$ \begin{split} c_t &= \theta c_{t-1} + \theta_g g_t \\ h_t &= \tanh(c_t) \end{split} $$
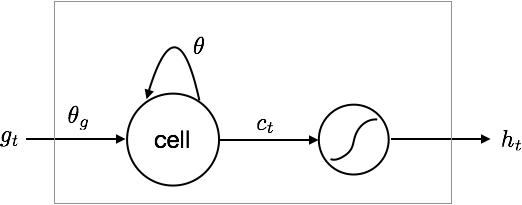
Long Short Term Memory (LSTM)¶
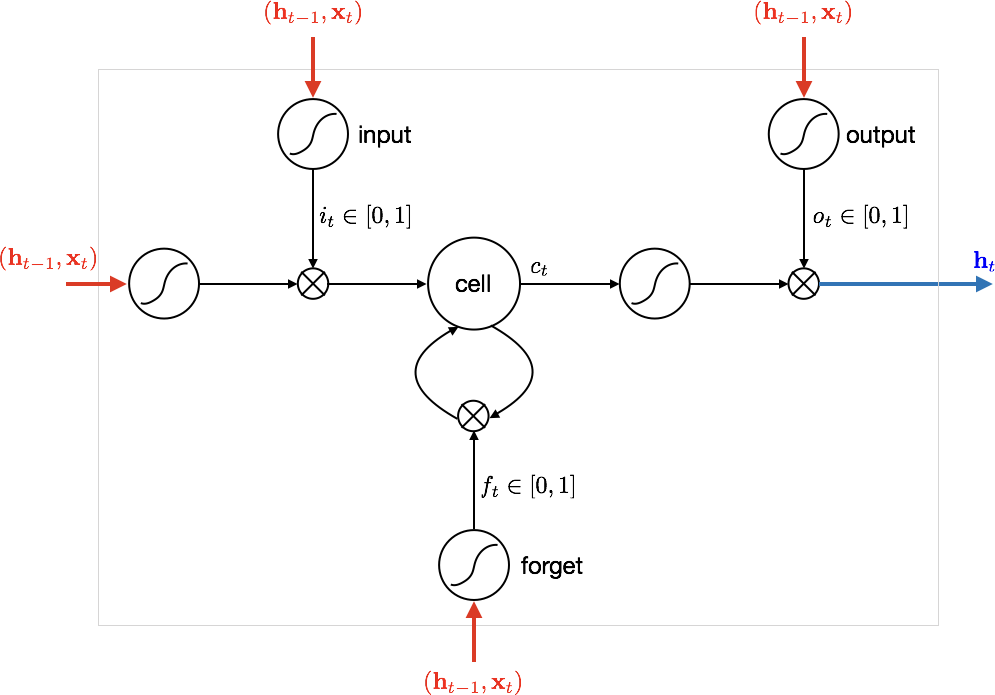
$\newcommand{\bi}{\mathbf{i}}$ $\newcommand{\bb}{\mathbf{b}}$ $\newcommand{\bc}{\mathbf{c}}$ $\newcommand{\bo}{\mathbf{o}}$ $\newcommand{\bo}{\mathbf{o}}$ $\newcommand{\bx}{\mathbf{x}}$ $\newcommand{\boldf}{\mathbf{f}}$
- Input gate: scales input to cell (write operation)
- Output gate: scales input from cell (read operation)
- Forget gate: scales old cell values (forget operation)
$$ \begin{split} \bi_t &= \text{sigmmoid}(\theta_{xi} \bx_t + \theta_{hi} \bh_{t-1} + \bb_{i}) \\ \boldf_t &= \text{sigmmoid}(\theta_{xf} \bx_t + \theta_{hf} \bh_{t-1} + \bb_{f}) \\ \bo_t &= \text{sigmmoid}(\theta_{xo} \bx_t + \theta_{ho} \bh_{t-1} + \bb_{o}) \\ \bg_t &= \tanh(\theta_{xg} \bx_t + \theta_{hg} \bh_{t-1} + \bb_{g}) \\ \bc_t &= \boldf_t \circ \bc_{t-1} + \bi_t \circ \bg_t \\ \bh_t &= \bo_t \circ \tanh(\bc_t) \end{split} $$
$\circ$ represent element-wise multiplication
RNN vs. LSTM¶
Check out the video at https://imgur.com/gallery/vaNahKE
Summary¶
- RNN
- Allow a lot of flexibility in architecture design
- Very difficult to train in practice due to vanishing and exploding gradients
- Control gradient explosion via clipping
- Control vanishing gradients via LSTMs
- LSTM
- A powerful architecture for dealing with sequences (input/output)
- Works rather well in practice
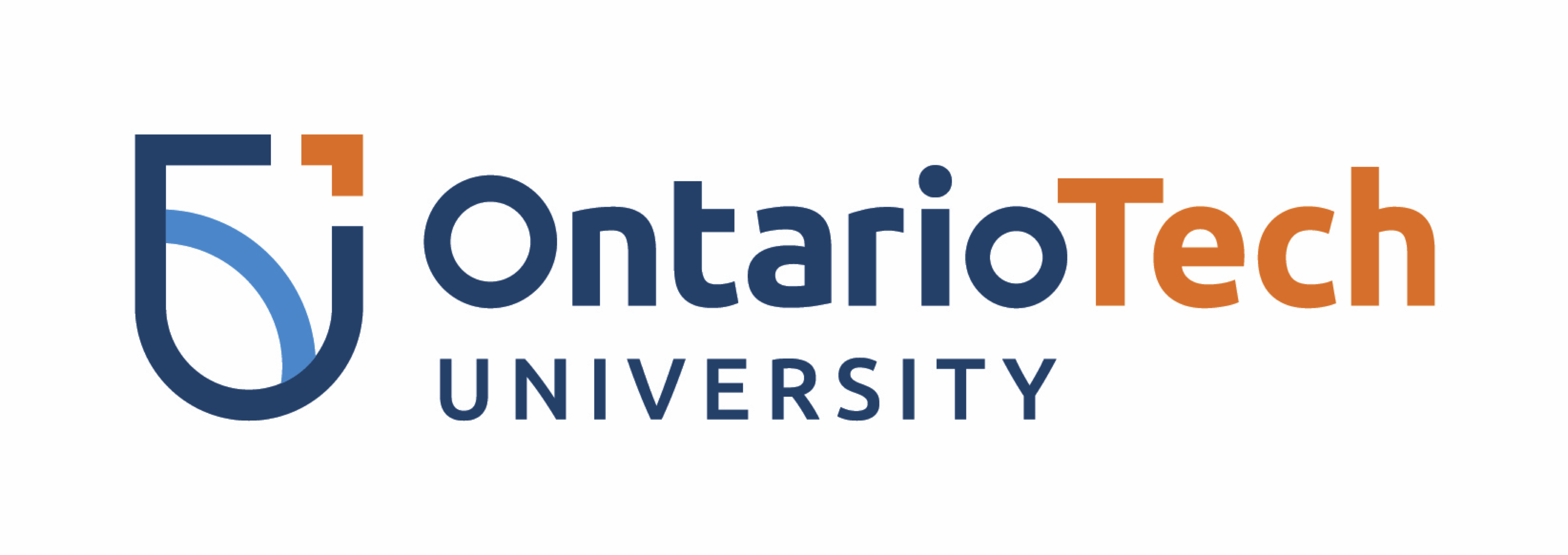